Tuesday 23 July 2019
Lupine Publishers: A Women’s Place is at the Heart of the Home | Lupi...
Lupine Publishers: A Women’s Place is at the Heart of the Home | Lupi...: Journal of Gynaecology | Lupine Publishers Opinion One of the most dangerous things happening right now is the breakup of t...
Friday 19 July 2019
Lupine Publishers | Current Trends in Computer Sciences & Application
Computer Science Research Journals | Lupine Publishers


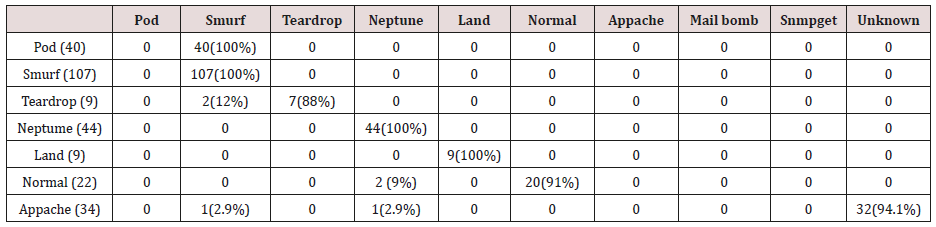
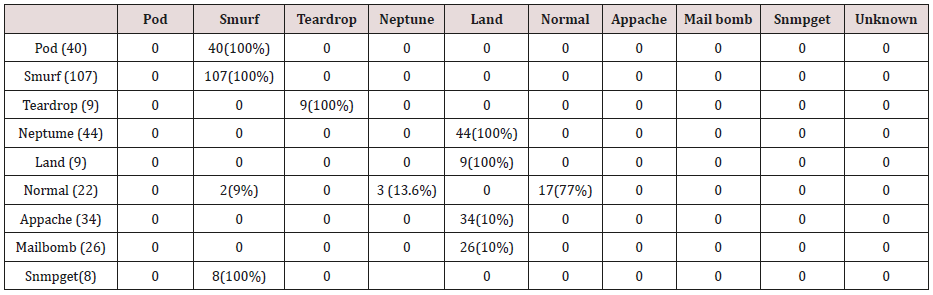
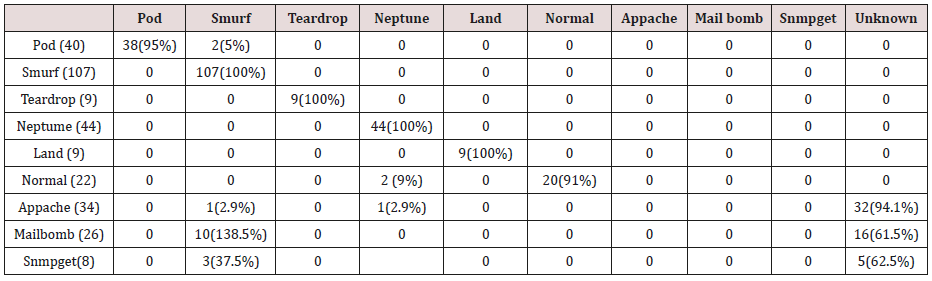
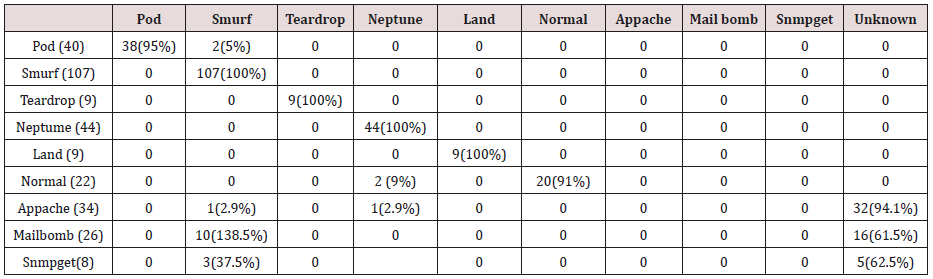
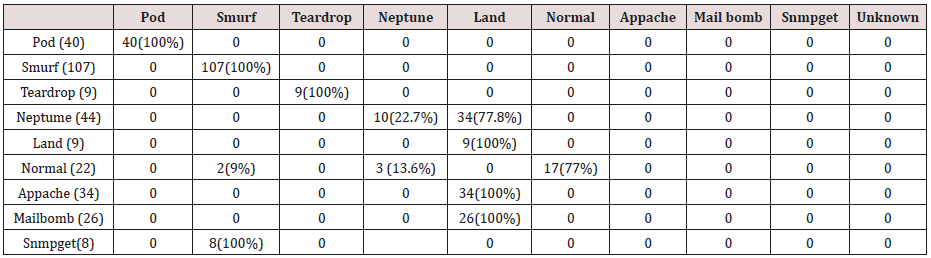
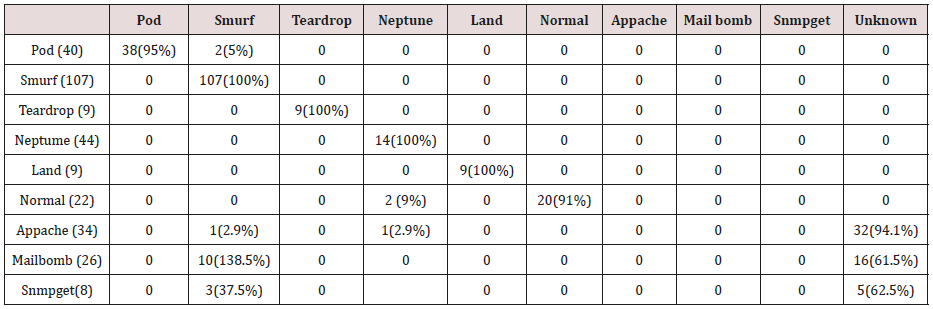
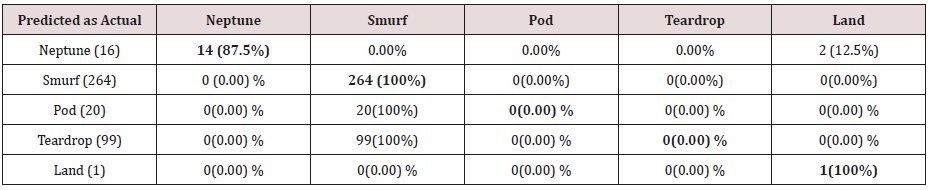
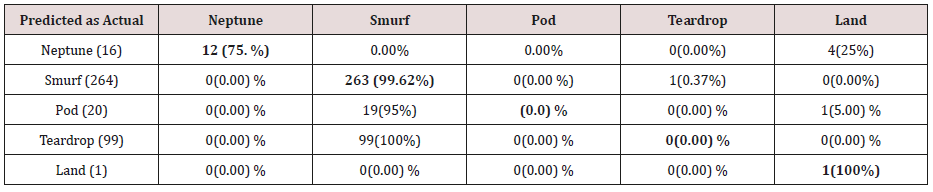
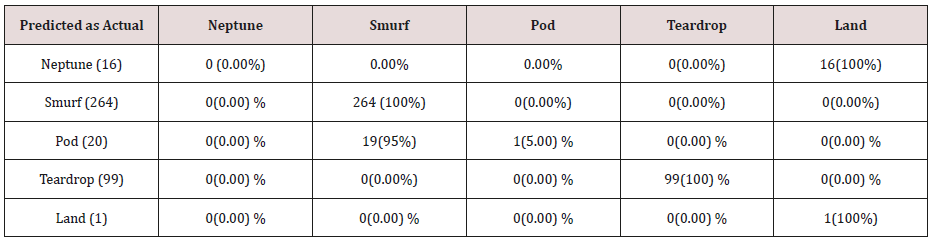




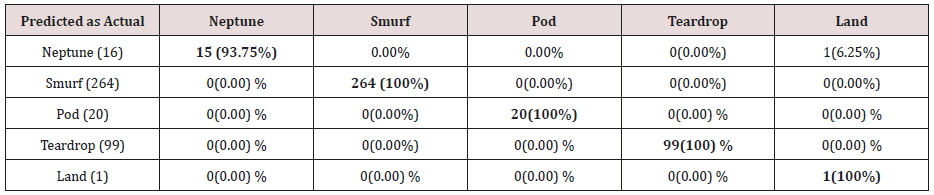


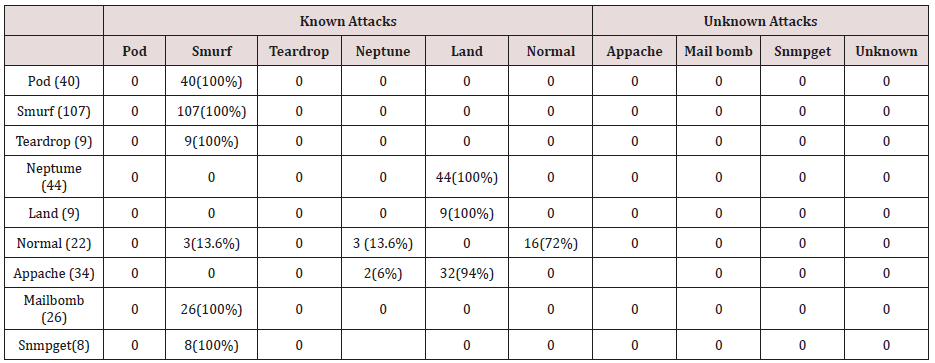
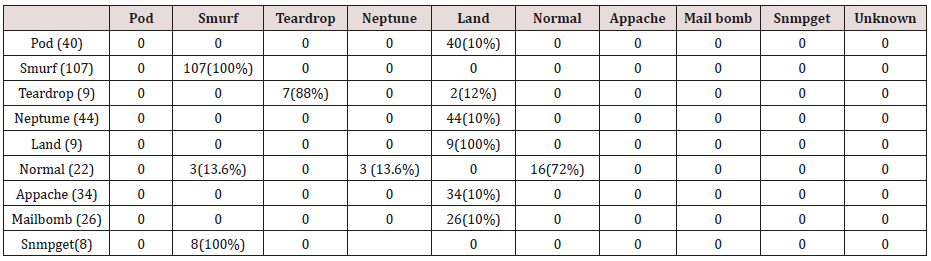
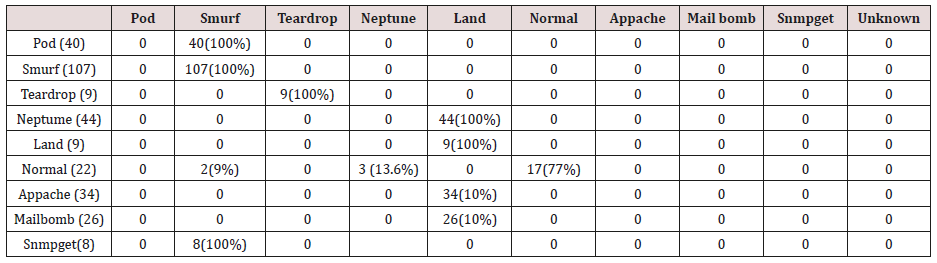

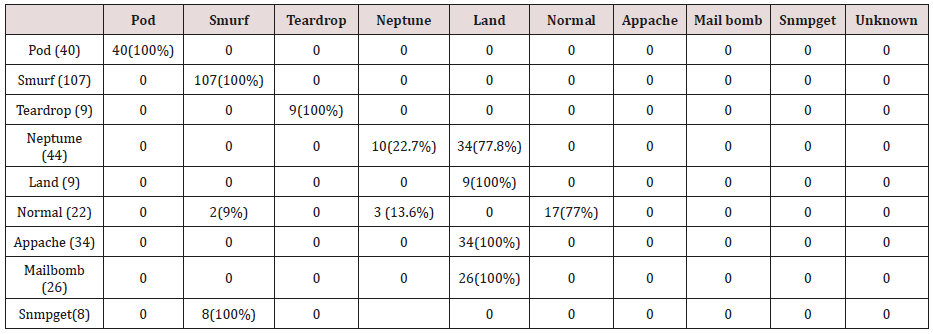
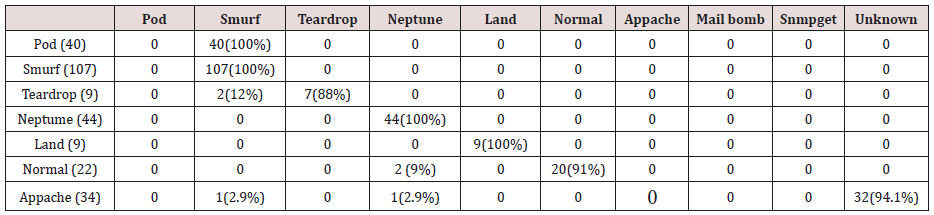
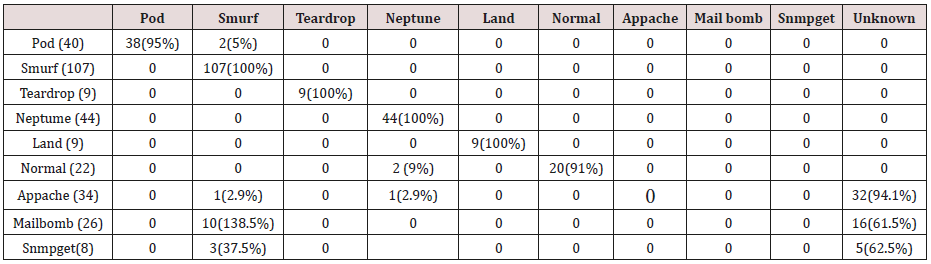
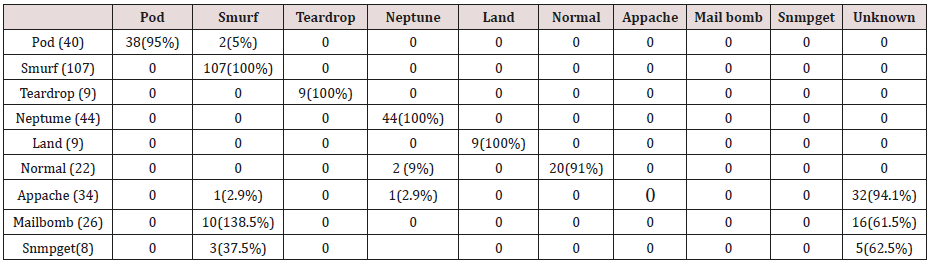
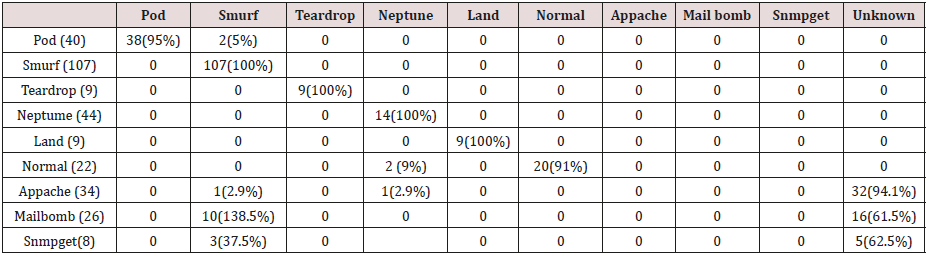
![]() |
Detecting Distributed Denial-of-Service DDoS Attacks |
Abstract
Since the number of damage cases resulting from distributed denial-of-service (DDoS) attacks has recently been increasing, the need for agile detection and appropriate response mechanisms against DDoS attacks has also been increasing. The latest DDoS attack has the property of swift propagation speed and various attack patterns. There is therefore a need to create a lighter mechanism to detect and respond to such new and transformed types of attacks with greater speed. In a wireless network system, the security is a main concern for a user.
Introduction
Security of information is of utmost importance to organization striving to survive in a competitive marketplace. Network security has been an issue since computer networks became prevalent, most especially now that internet is changing the face of computing. As dependency on Internet increases on daily basis for business transaction, so also is cyber-attacks by intruder who exploit flaws in Internet architecture, protocol, operating systems and application software to carry out their nefarious activities Such hosts can be compromised within a short time to run arbitrary and potentially malicious attack code transported in a worm or virus or injected through installed backdoors. Distributed denial of service (DDoS) use such poorly secured hosts as attack platform and cause degradation and interruption of Internet services, which result in major financial losses, especially if commercial servers are affected (Duberdorfer, 2004).
Related Works
Brignoli et al. [1] proposed DDoS detection based on traffic selfsimilarity estimation, this approach is a relatively new approach which is built on the notion that undisturbed network traffic displays fractal like properties. These fractal-like properties are known to degrade in presence of abnormal traffic conditions like DDoS. Detection is possible by observing the changes in the level of self-similarity in the traffic flow at the target of the attack. Existing literature assumes that DDoS traffic lacks the self-similar properties of undisturbed traffic. The researcher shows how existing bot- nets could be used to generate a self-similar traffic flow and thus break such assumptions. Streilien et al,2005. Worked on detection of DoS attacks through the polling of Remote Monitoring (RMON) capable devices. The researchers developed a detection algorithm for simulated flood-based DoS attacks that achieves a high detection rate and low false alarm rate.
Yeonhee Lee [2] focused on a scalability issue of the anomaly detection and introduced a Hadoop based DDoS detection scheme to detect multiple attacks from a huge volume of traffic. Different from other single host-based approaches trying to enhance memory efficiency or to customize process complexity, our method leverages Hadoop to solve the scalability issue by parallel data processing. From experiments, we show that a simple counterbased DDoS attack detection method could be easily implemented in Hadoop and shows its performance gain of using multiple nodes in parallel. It is expected that a signature-based approach could be well suited with Hadoop. However, we need to tackle a problem to develop a real time defense system, because the current Hadoop is oriented to batch processing.
Proposed System Architecture of Intrusion Detection Based on Association Rule
The structure of the proposed architecture for real time detection of Dos instruction detection via association rule mining, it is divided into two phases: learning and testing. The network sniffer processed the tcpdump binary into standard format putting into learning, during the learning phase, duplicate records as well as columns with single same data were expunge from the record so as to reduce operational. Another table Hashmap was created by the classification model to keep track of the count of various likely classmark that can match the current read network traffic, this table will be discarded once the classmark with highest count had been selected. Depicted in Table 1 is the Association rule classifier algorithm (Tables 2-4).
System Implementation
This chapter presents implementation of Association rule classifier model, documentation of the designed system and the user interfaces. The software and hardware requirement needed for the system and also the testing of the system for verification and validation of functions, as well as the result [3-10].
Interface Design
Start Page: This page is the first page that is seen when the application is executed. The option button enables the use of already generated rules to be reused for classification of another file. If the check box is unselected, the option of selecting a folder containing already generated rule is enabled. Furthermore, select the source file and then the new file to classify. The page is as shown below (Figure 1).
Creating Folder: The first step is to create a folder where the rules will be saved, and also the numbers for generating the rules will be saved. This operation is allowed only if the check box on the form is checked, if there is an error with the folder creation process, an error message will be displayed. The open button for the file name is enabled if the folder is created successfully. The interface for the creation of folder is shown below (Figure 2).
Source File: The source of data for generating the rule is the next requirement. The open button closes to the Mine button enable you to specify the file containing the data from which the rules will be generated. Before the rules are generated from the file, the size of the file is calculated to obtain the number of combination(arrangement) required to generate the rule. The selected source file is seen below (Figure 3).
a) Mining File: The selected source file is mined to extract the rules needed for classification. Depending on the size of the file, it could take a while to complete. On completion, a message dialog is displayed, as shown below (Figure 4).
b) File Classification: After obtaining all the rules from the source file, the open button for selecting a data file to classify is enabled. A file can be classified based on the rules generated. The selected file can be obtained using the open button, as shown below (Figure 5).
c) Generate Result: Click on the start button to begin the generation of output or result from the selected file to classify, based on the rules generated. The output or result is saved in the folder called result within the folder specified above. Depending on the size of the file to classify, the output might take a while. On completion of the classification, a dialog appears to signify the completion of the classification. This is shown in Figure 6.
d) Exit Program: Click the Exit button to exit or terminate the application (Figure 7).
Experimental Setup and Results
The training dataset consisted of 37,079 records, among which there are 99(.266%) teardrop, 36,944 (99.66%) smurf, 20(0.05%) pod, 15(0.04%) neptume and 1(0.026%) land connections. The training dataset use for testing is made up of 400 records out of which there are 98(24.5%) teardrop, 266(66.5%) smurf, 2 (5%) pod, 15(3.75%) neptume and 1 (0.025%) land while the test dataset is made up of 300 records out of there are 40 (13.3%) Pod, 107 (35.6%) smurf, 9(3%) teardrop, 43(14.3%) neptune (14.3%), 9(3%) land, 33(11%) apache2, 21(7%) normal, 25(8.3%) mailbomb and 8(2.6%) snmpgetattack [11-20].
The test and training data are not from the same probability distribution. In each connection are 20 attributes out of 41 attributes describing different features of the connection (excluding the label attribute)
The experiment with association rule classification are divided into two major phases, in the first phase, rules were generated for each, (and combinations of attribute) of the traffic network dataset using the two association rule indices; Confidence and support, in the second phase, the rule generated in the first phase were then pruned to remove irrelevant rules so as to improve the classification process, the pruning process include;
i. Removal of all rules with confidence less than 50%
ii. Removal of all duplicate rules
iii. All identical rules pointing to difference attacks were also removed
iv. All one attribute rules were not considered for classification
ii. Removal of all duplicate rules
iii. All identical rules pointing to difference attacks were also removed
iv. All one attribute rules were not considered for classification
Both the initial rules generate, and pruned rules were then used to classify the training set as well as testing data set.
Results
Tables 5-14 shows the confusion matrix obtained Association rule mining with 20 attributes.
Table 5: Confusion matrix obtained from one attribute combination from test dataset (unprune rules).

Table 7: Confusion matrix obtained from one and two attribute combination from test dataset (unprune rules).

Table 8: Confusion matrix obtained from one and two attribute combination from test dataset (prune rules).
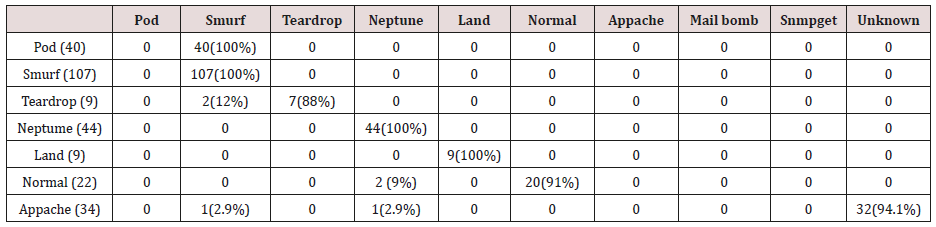
Table 9: Confusion matrix obtained from one, two and three attribute combination from test dataset (unprune rules).
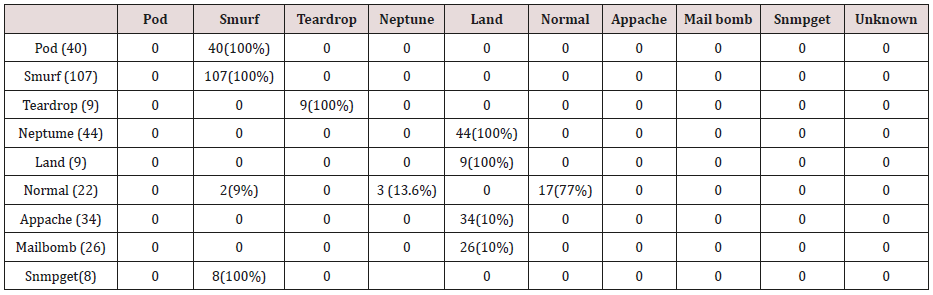
Table 10: Confusion matrix obtained from one, two and three attribute combination from test dataset (prune rules).
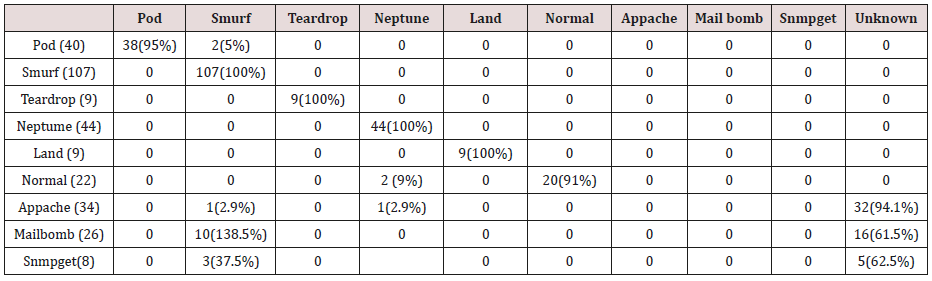
Table 12: Confusion matrix obtained from one, two, three and four attribute combination from test dataset (prune rules).
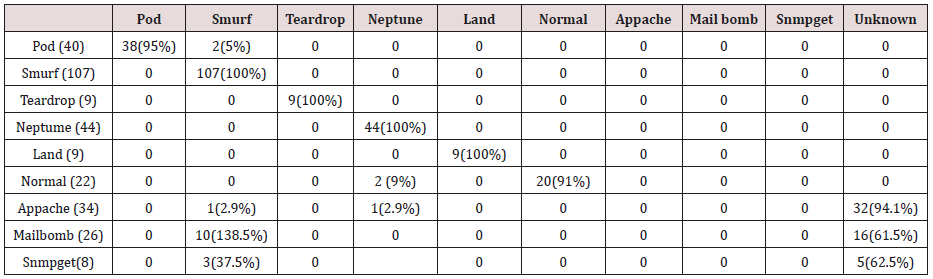
Table 13: Confusion matrix obtained from one, two, three, four and five attribute combination from test dataset (unprune rules).
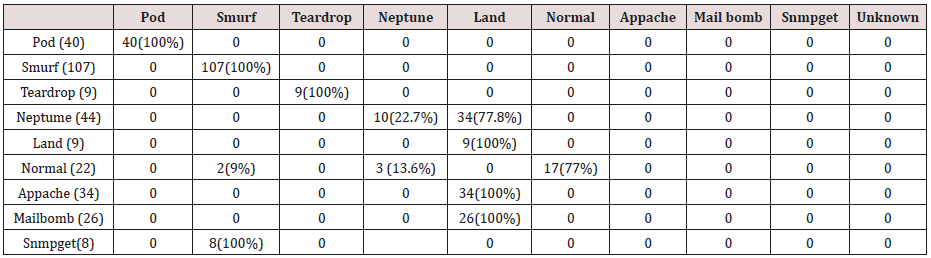
Table 14: Confusion matrix obtained from one, two, three, four and five attribute combination from test dataset (prune rules).
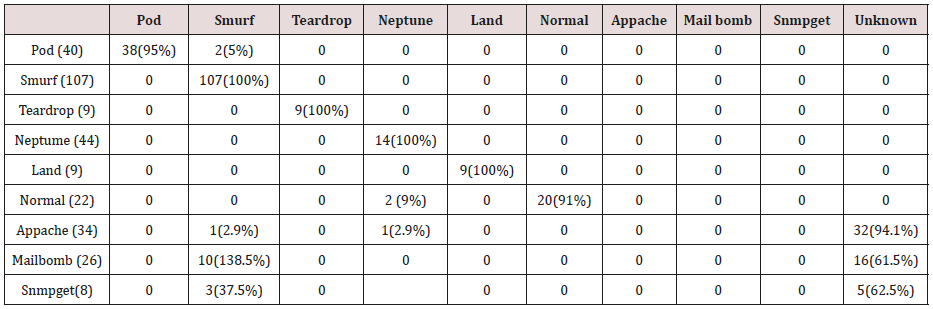
Discussion
The results in Tables 15-19, were obtained from classification of training data set with raw unprune rule set, from the tables, the degree of accuracy of classification of smurf attack ranges between 99.6% to 100%. Pod attacks classification could not be classified correct by the classification model, about 95% pod attacks were classified as smurf attacks, while the rest were classified as Pod. 98% of teardrop and 100% of land attacks were also correctly classify, while less than 20% of Neptune attacks were classified correctly using rules based on 0ne, two and three combinational attributes, rules based on four and five combinational attributes has better performance of classification of Neptune attacks (65%) than one – three attributes combination. Table 20 shows the summary of all the attacks correctly classified in Tables 15-19.
Table 15: Confusion matrix obtained from one attribute combination from training dataset (unprune rules).
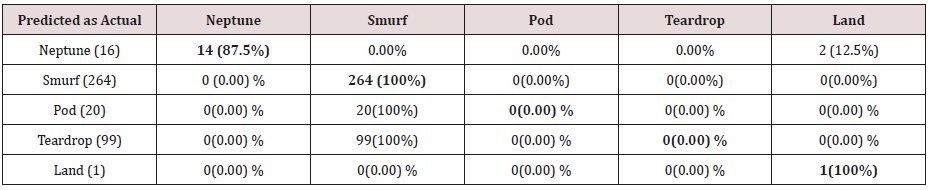
Table 16: Confusion matrix obtained from one and two attribute combination from training dataset (unprune rules).
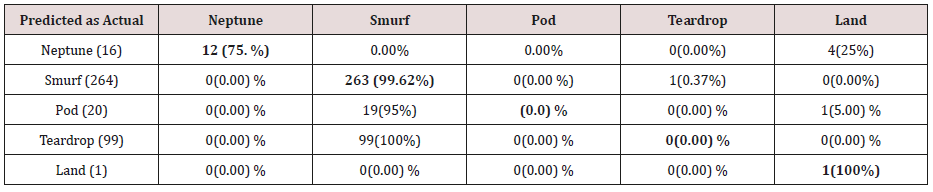
Table 17: Confusion matrix obtained from one, two and three attribute combination from training dataset (unprune rules).
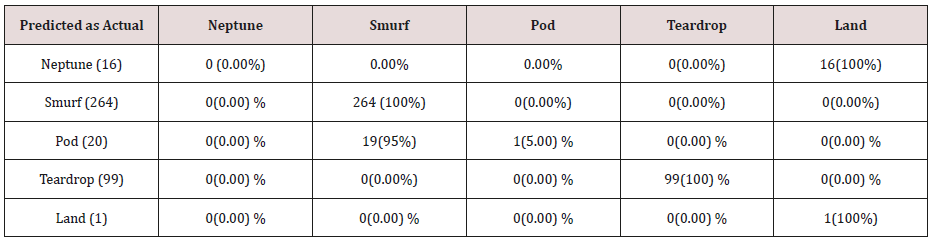
Table 18: Confusion matrix obtained from one, two, three and four attributes combination from training dataset (unprune rules).

Table 19: Confusion matrix obtained from one, two, three, four and five attributes combination from training dataset (unprune rules).

Table 21: Confusion matrix obtained from one attribute combination from training dataset (prune rules).

Table 22: Confusion matrix obtained from one and attribute combination from training dataset (prune rules).

Table 23: Confusion matrix obtained from one, two and three attribute combination from training dataset (prune rules).
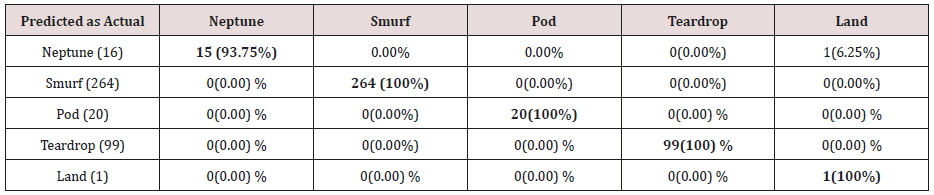
Table 24: Confusion matrix obtained from one, two, three and four attributes combination from training dataset (prune rules).

Table 25: Confusion matrix obtained from one, two, three, four and five attributes combination from training dataset (prune rules).

The results in Tables 21-25, were obtained from classification of training data set with pruned rule set, the pruned rule set gives a better results than the unpruned data set. All the attacks types except Neptune and pod were correctly classified (100%) for all the rules categories, pod was 90% correctly classifier with single attributes rules and 100% correctly classified with other four categories of rules. Neptune recorded 100% correct classification for 4 and 5 attributes combinational rules, 93% correct classification for 2 and 3 attributes combinational rules and 69% correctly classified for one attributes rules. Table 26 shows the summary of all the attacks correctly classified in Tables 21-25.
Implementation with Test Data
The association rule classifier was tested with test data that did not belong to the same network with the training dataset, there are three (3) (Appache, Mail bomb, Snmpget attacks in the test data that were not present in the training set. (Figures 1-7) shows the confusion matrix table obtained from the association rule classification of the test data
Discussion
Table 27: Confusion matrix obtained from one attribute combination from test dataset (unprune rules).
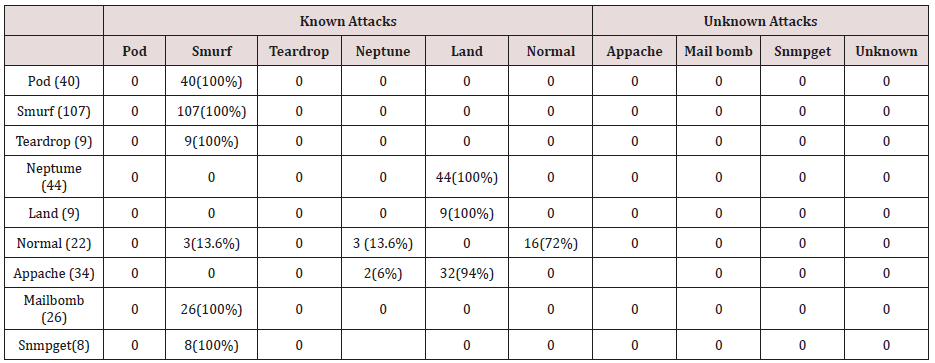
Table 28: Confusion matrix obtained from one and two attribute combination from test dataset (unprune rules).
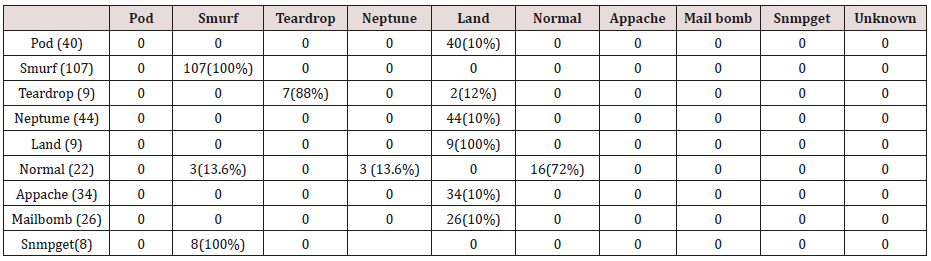
Table 29: Confusion matrix obtained from one, two and three attribute combination from test dataset (unprune rules).
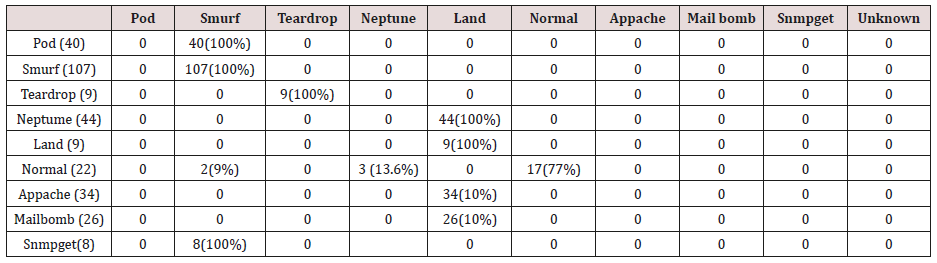
Table 30: Confusion matrix obtained from one, two, three and four attribute combination from test dataset (unprune rules).

Table 31: Confusion matrix obtained from one, two, three, four and five attribute combination from test dataset (unprune rules).
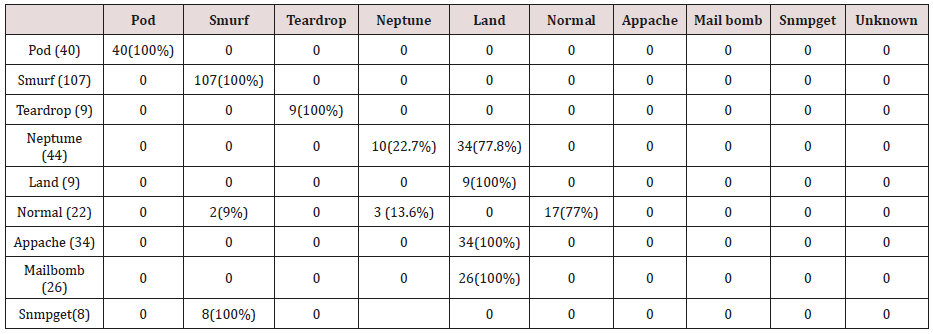
The results in Tables 27-31 were obtained from classification of test data set with the raw unpruned rules. From the tables, Pod attacks were classified Teardrop and smurf attacks. Smurf and Teardrop attacks were 100% and 88% classified correctly respectively, all Neptume attacks were classified as Land attacks, all Land attacks were correctly classified, between 77% and 90% of Normal traffic were classified correctly. 94% and 6% of Appache attack were classified as Land and Neptume attack respectively. Smnpget attacks were classified as smurf and Teardrop attacks.
Table 33: Confusion matrix obtained from one and two attribute combination from test dataset (prune rules).
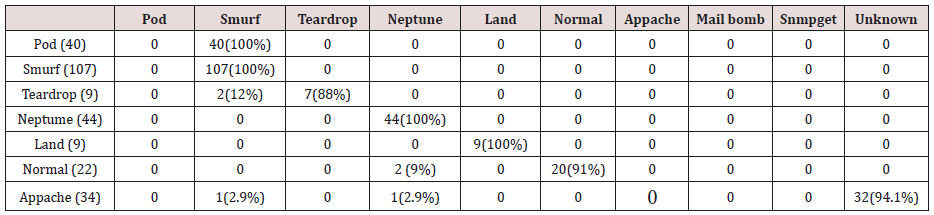
Table 34: Confusion matrix obtained from one, two and three attribute combination from test dataset (prune rules).
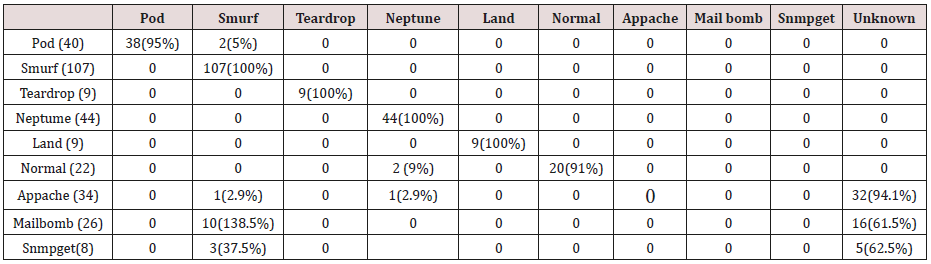
Table 35: Confusion matrix obtained from one, two, three and four attribute combination from test dataset (prune rules).
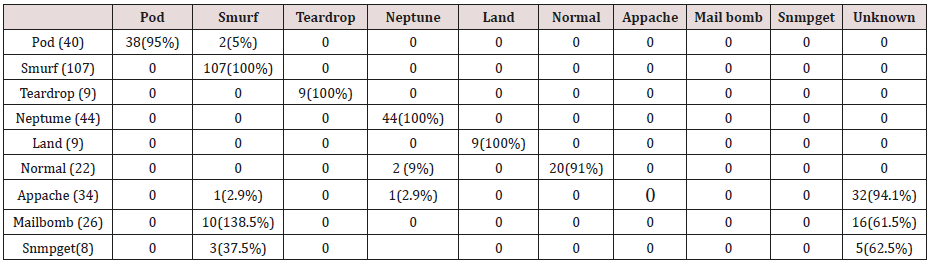
Table 36: Confusion matrix obtained from one, two, three, four and five attribute combination from test dataset (prune rules).
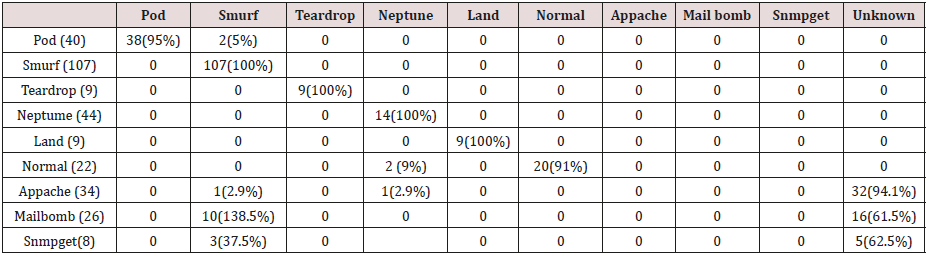
The results in Tables 32-36 were obtained from classification of test data set with the raw pruned rules. 3,4, and 5 attributes rules classified Pod, Smurf Teardrop, Neptume and Land attacks correctly. Appache, mailbomb and snmpget attacks were classified as either Unknown, Smurf or Teardrop attacks. Table 37 shows the summary of all the correctly classified attacks. All the attacks present in the test dataset which were not used for training of the Association rule classifier were classified as attacks with unprune rule, the pruned rule classified these attacks as unknown attacks. Tables 38 & 39 below shows how they were classified [21-46].
Conclusion
The need for effective and efficient security on our system cannot be over-emphasized. This position is strengthened by the degree of human dependency on computer systems and the electronic superhighway (Internet) which grows in size and complexity on daily basis for business transactions, source of information or research. Association Rule methods of improving intrusion detection systems based on machine learning techniques were described and implemented on Intel Duo-core, CPU 2.88GHz, 1024MB RAM using Java programming language.
The work is motivated by increasing growth in importance of intrusion detection to the emerging information society. The research work provided background detail on intrusion detection techniques, and briefly described intrusion detection systems. In this research, an Association rule-based algorithm, was newly developed for mining known known-patterns. The results of the developed tools are satisfactory though it can be improved upon. These tools will go a long way in alleviating the problems of security of data by detecting security breaches on computer system.
For more Lupine Publishers Open Access Journals Please visit our website: www.lupinepublishersgroup.com/
For more Computer Science Journal Please click here: https://lupinepublishers.com/computer-science-journal/index.php
Subscribe to:
Posts (Atom)
Wishing you a Magical and Blissful Holiday
Take a leap of faith and begin this wondrous new year by believing. Happy New Year!

-
Lupine Publishers: List Of Open Access Journals in Lupine Publishers : agriculture open access journals Add caption Journal of Chem...
-
Lupine Publishers: Effect of Interaction Between Ag Nanoparticles and... : Lupine Publishers- Environmental and Soil Science Abstract ...
-
Lupine Publishers: Lupine Publishers | Fibonacci Circle in Fashion De... : Lupine Publishers | Journal of Textile and Fashion Designing ...